
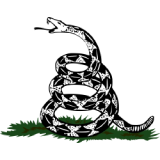
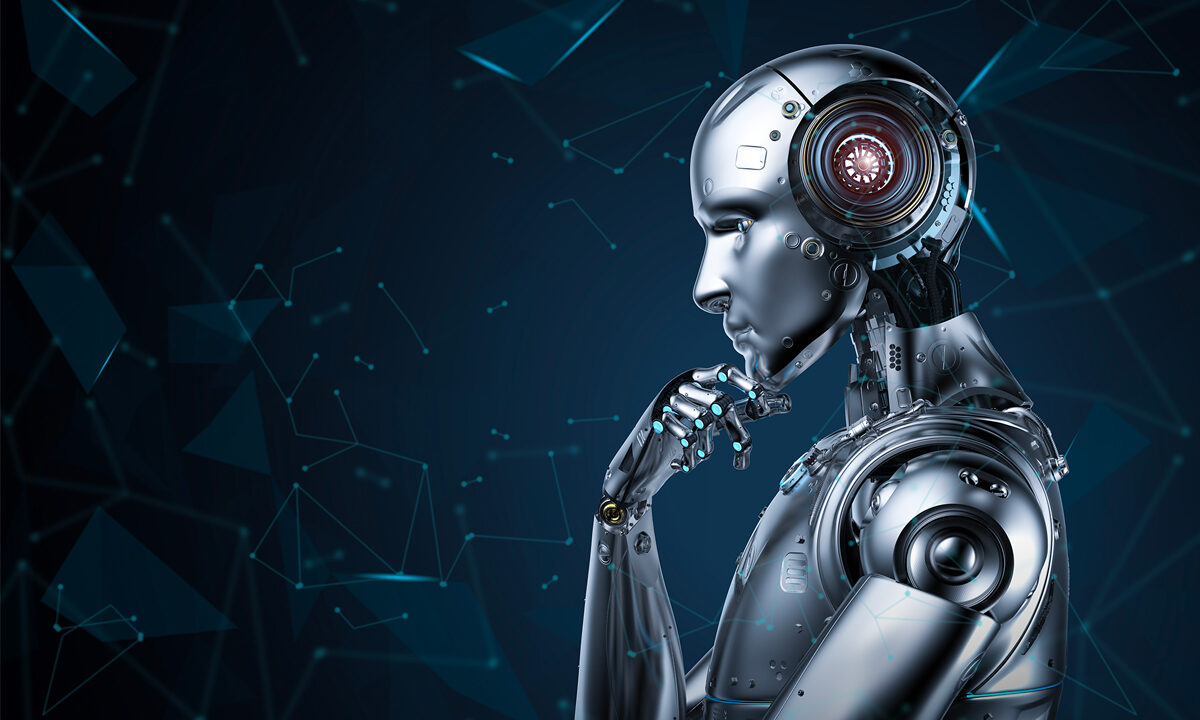
Artificial intelligence (AI) is making headlines, and the reviews are mixed.
Though the United States leads the world in AI investment, Americans remain skeptical. According to a global survey (pdf) by Ipsos, “Only 35 percent of sampled Americans agreed that products and services using AI had more benefits than drawbacks.” Among surveyed countries, America had one of the lowest percentages of those who agreed with the statement.
Private industry has edged out academia in producing state-of-the-art AI systems; at the same time, the number of incidents of ethical misuse of AI has increased dramatically—from 10 in 2012 to over 250 in 2021.
The field is growing in what could be called a “Wild West” of AI. According to Stanford University’s AI Index Report, “AI has moved into its era of deployment; throughout 2022 and the beginning of 2023, new large-scale AI models have been released every month.” These models include Stable Diffusion, Whisper, DALL-E 2, and the ubiquitous ChatGPT. The area with the most investment? Health care.
The possibilities of AI in health care seem endless. Still, whether AI offers promise or peril remains in question.
Though there’s a lot of buzz about AI, artificial intelligence isn’t new. Theoretical work on “machine learning” is credited to Alan Turing’s research beginning in 1935. The term “artificial intelligence” appeared in the early 1950s and was used in a 1955 proposal for a summer research project at Dartmouth College (pdf). The following summer, 10 scientists met to study whether machines could simulate human learning and creativity. Their findings would change the course of science.
A basic definition of AI is “software used by computers to mimic aspects of human intelligence.” Under the umbrella of AI are specialties like “machine learning” and “deep learning” that can make decisions without humans.
Scientists have utilized AI in medical research since the 1970s. The technology can analyze large amounts of data to provide personalized treatment recommendations and identify patterns and risks that might not be immediately apparent to the human eye. In the right hands, AI could revolutionize medical care.
A Massachusetts Institute of Technology (MIT) research team partnered with Massachusetts General Hospital (MGH) in Boston and Chang Gung Memorial Hospital in Taiwan to create an AI tool that assesses lung cancer risk. Introduced in January 2023, “Sybil” uses a single low-dose CT scan to predict cancer that will occur within one to six years, with remarkably high accuracy—up to 94 percent in a clinical trial. In the Journal of Clinical Oncology, researchers summed up Sybil’s early success: “Sybil was able to forecast both short-term and long-term lung cancer risk” and “maintained its accuracy across diverse sets of patients from the United States and Taiwan.”
Lung cancer is the deadliest cancer in the world “because it’s relatively common and relatively hard to treat, especially once it has reached an advanced stage,” stated Dr. Florian Fintelmann, a radiologist physician-scientist at MGH, associate professor of radiology at Harvard Medical School, and part of the research team. Fintelmann noted that the five-year survival rate is 70 percent for early detection but drops to 10 percent for advanced detection.
Sybil’s ability to predict cancer outcomes can lead to more widespread screening, especially in underserved populations. This is in keeping with guidance from the U.S. Food and Drug Administration (FDA) on improved clinical trial enrollment among members of minority communities.
While Sybil awaits approval by the FDA, 521 AI algorithms have already been approved.
Three-quarters of these are in medical imaging, and another 56 are cardiology-related applications.
Because machine learning evolves with new data, going forward, the FDA will require applications for AI to include predetermined change control plans (PCCPs). Accordingly, it has recently issued a draft guidance on PCCPs.
This is to ensure AI “can be safely, effectively, and rapidly modified, updated, and improved in response to new data,” Brendan O’Leary, deputy director of the Digital Health Center of Excellence in the FDA’s Center for Devices and Radiological Health, said in a statement.
If the guidance is approved, developers can update AI devices without submitting a new application to the FDA.
It is likely that, even with the FDA’s increased data requirements, there will be no slowing the development of AI devices and algorithms.
AI was created to imitate how humans think, reason, and solve problems. Humans are fallible and have biases; however, AI may not be better.
AI’s judgment is based on the data it is fed. “Data bias” occurs when an algorithm is trained with poor or incomplete data, which leads to faulty predictions.
While numerous studies have claimed that AI could assess skin cancer more accurately than human doctors could, one group of researchers decided to challenge an AI application.
The researchers began with 25,331 training images from two datasets—one from Vienna and the other from Barcelona—including eight skin diseases. Then, they added images—from Turkey, New Zealand, Sweden, and Argentina—that had not been used in the training data and included additional skin diseases.
AI misclassified nearly half (47.1 percent) of the images from outside the training datasets. According to the researchers, this would “lead to a substantial number of unnecessary biopsies if current state-of-the-art AI technologies were clinically deployed.”
Even the most promising AI requires real-world clinical trials before it can be adopted.
How do AI developers measure the success of their algorithms? Typically, they conduct studies with datasets from the past.
As Eugenio Santoro of the Mario Negri Institute of Pharmacological Research wrote, “Many of these are retrospective and based on previously assembled datasets, while few are prospective ones conducted in real clinical settings, and very few are those based on randomized controlled clinical trials.”
Humans can be influenced by computer- or AI-generated data—even when those data are incorrect. So to what extent, if any, could AI bias medical professionals?
In an experiment conducted by German and Dutch researchers, 27 radiologists read 50 mammograms. The radiologists were given (fake) AI-generated categorizations for the mammograms, half of which were incorrect. (Categorizations suggest the next steps in treatment.) The radiologists were unwittingly influenced by the AI-generated assessments: “Experienced radiologists, those with more than 15 years of experience on average, saw their accuracy fall from 82 percent to 45.5 percent when the purported AI suggested the incorrect category.”
The researchers wrote that safeguards are needed to avoid this kind of bias, and one of the safeguards is that we should know “the reasoning process” of AI—that is, what takes place in the so-called “black box.”
The theoretical place where all of what goes on between input (data) and output is called a “black box.”
Because machine learning can teach itself, some of what’s happening inside the black box remains mysterious, even to its creators.
In AI, accuracy is everything. The prevailing idea is that to achieve this accuracy, AI must be complicated and uninterpretable. However, scientists are beginning to challenge that notion.
According to a paper published in the Harvard Data Science Review, “the so-called accuracy–interpretability tradeoff is revealed to be a fallacy: More interpretable models often become more (and not less) accurate.” Furthermore, the authors explained, “When scientists understand what they are doing when they build models, they can produce AI systems that are better able to serve the humans who rely upon them.”
The black box also contributes to distrust, mainly due to its “highly opaque nature or inexplicability.” In a white paper published by the Italian Society of Medical and Interventional Radiology this May, the authors noted, “Even experts at the highest level may struggle to fully understand the so-called ‘black-box’ models.”
The white paper authors refer to “explainable AI” as an important aspect of AI adoption, calling for developers to move from “black box” models to “glass box” models.
It’s not just what happens inside the box that is hidden; the training data fed into AI algorithms might surprise you.
How AI learns is modeled after how humans learn. We learn, from infancy, from the people around us. Likewise, AI does not exist in a vacuum. Before it can work its magic, it needs data.
And that data comes from you and me.
Our data are collected in myriad ways. If you use a health app online or wear a “smart” device, your fitness tracker may be keeping track of every step you take and transmitting that data to a company that bundles and sells them. If you check the local weather on a smartphone, chances are you have turned on your phone’s location tracking. Did you know that the app tracks everywhere you go and how much time you spend there and can infer from that data what religion you practice, whether or not you vote, and even your age?
What about medical data? Most Americans are familiar with the Health Insurance Portability and Accountability Act (HIPAA), which protects our privacy related to health information.
However, there are gaps in HIPPA. “Numerous apps and websites outside the scope of HIPAA’s narrow ‘covered entities’ are entirely free to legally collect, aggregate, and sell, license, and share Americans’ health information on the open market,” Justin Sherman, senior fellow and research lead at Duke University Sanford School of Public Policy’s data brokerage project, explained in his written testimony to the U.S. House Committee on Energy and Commerce.
Some of the data come directly from hospitals. According to VentureBeat, “Google maintains a 10-year research partnership with the Mayo Clinic that grants the company limited access to anonymized data it can use to train algorithms.”
In what it calls “a move to democratize research on artificial intelligence and medicine,” Stanford University maintains “the world’s largest free repository of AI-ready annotated medical imaging datasets.”
“What drives this technology, whether you’re a surgeon or an obstetrician, is data,” stated Matthew Lungren, co-director of Stanford’s Center for Artificial Intelligence in Medicine & Imaging (AIMI) and an assistant professor of radiology at Stanford. “We want to double down on the idea that medical data is a public good and that it should be open to the talents of researchers anywhere in the world.”
Is that really what we want—for our medical data to be a “public good”?
Who could have imagined that AI would go from science fiction to dinner-table conversation in what seems like the blink of an eye? Certainly, AI-based tools like ChatGPT have made AI widely accessible.
While some call for a moratorium on AI development, others quickly advance. Predicting what the next five years will look like in AI is nearly impossible. But don’t ask a robot; it can’t predict the future.
At least, not yet.